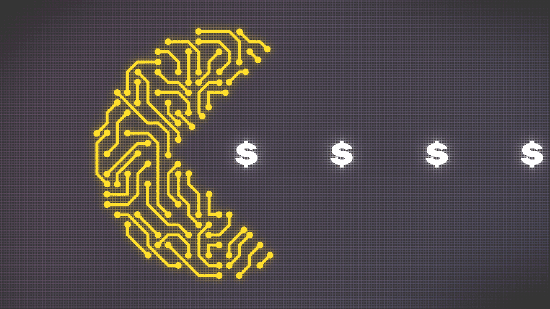
The cost of building artificial intelligence is doubling every nine months, a new study shows. Here's why.
Artificial intelligence executives have big plans—and they’re not cheap. In a recent interview with TIME, Dario Amodei, CEO of AI company Anthropic predicted that the cost to develop the next generation of AI systems that will be released later this year would be around $1 billion. This trend suggests that the generation after that would cost more like $10 billion.
Amodei is not the only one preparing for a spending spree. Microsoft and OpenAI are reportedly planning to build a $100 billion supercomputer to build and run AI models. Asked about this plan, Google DeepMind CEO Demis Hassabis said that his company will invest more over time.
[time-brightcove not-tgx=”true”]Read More: Inside Anthropic, the AI Company Betting That Safety Can Be a Winning Strategy
In a new study released Monday, two researchers from Stanford University and three researchers from Epoch AI, a nonprofit research institute that focuses on predicting how AI will develop, published the most thorough analysis yet of how the cost to train the most capable AI systems has evolved over time, and what is driving the spiraling costs of the AI arms race between technology companies. Their results suggest that the costs of training the most advanced AI systems has been increasing for years as a result of the growing amount of computational power being used to train those systems, and that employee compensation is also a significant contributor to the cost of AI.
“The cost of the largest AI training runs is growing by a factor of two to three per year since 2016, and that puts billion-dollar price tags on the horizon by 2027, maybe sooner,” says Ben Cottier, a staff researcher at Epoch AI who led the study. This will mean only very well-funded companies will be able to compete, cementing the power of already powerful firms, he warns.
The cost of computation
To calculate the cost of the computational power required to train a given AI model, the researchers at Epoch AI took historical data on the cost to purchase the specialized semiconductor chips required, and then depreciated this figure over the time that the chips were required to run to train the AI model.
The researchers found that the cost of the computational power required to train the models is doubling every nine months. This is a prodigious rate of growth—at this rate, the cost of the hardware and electricity needed to build cutting-edge AI systems alone would be in the billions by later this decade, without accounting for other costs such as employee compensation.
However, the plans touted by Amodei and others outstrip even this rapid rate of growth. Costs could well increase beyond the historical rate for the next couple of years, before settling back down to the original trend in the longer term, says Cottier.
Top salaries
Accessing and powering the required computational horsepower is just part of the cost involved in developing the most sophisticated AI systems. Companies must also pay the researchers developing the algorithms. To estimate labor costs, the researchers at Epoch AI took the number of researchers who developed a given model as indicated by the number of co-authors on the paper announcing its release, and multiplied this number by an estimate for the average compensation of an AI researcher and an estimate for the time the researchers spent on developing the AI model.
They estimated labor costs for four AI models—OpenAI’s GPT-3 and GPT-4 models, a replication of GPT-3 developed by researchers at Meta named OPT-175B, and Google DeepMind’s Gemini Ultra 1.0—and found that employee compensation ranged from 29% to 49% of the total cost of development.
While a lot of discussion has focused on the escalating costs of accessing the specialized semiconductor chips required to train and run advanced AI systems—Amodei has attributed his astronomical projections of future costs to chips—Epoch AI’s results suggest that compensation is also a significant cost driver. However, if companies continue to train AI models with ever-greater amounts of computational power, Cottier expects labor costs to shrink as a proportion of total costs.
Winners take all
It’s not clear whether the trend documented in the study will hold. The push to build more computationally intense AI systems could be bottlenecked by the intense energy requirements of the largest clusters of semiconductor chips or by a lack of training data. Some commentators argue that it won’t make commercial sense for companies to continue training larger models in future, given the hefty expense and marginal incremental benefits of additional scale.
But if the trend does hold, only very well-resourced organizations will be able to keep pace. That includes tech giants—Google, Amazon, Microsoft, and Meta—and smaller companies backed by the tech giants, such as OpenAI and Anthropic, and a few other well-funded groups such as the U.A.E government-funded Technology Innovation Institute. Even within this narrow set of competitors, there are signs of an impending consolidation. In March, Inflection AI was “eaten alive” by its biggest investor, Microsoft, with most of its leadership team and employees joining the tech giant.
Given that such large investments are likely to produce remarkably capable AI systems, the paper’s authors warn that the “concentration of such a powerful technology among a few key players raises questions about responsible development and deployment. Both AI developers and policymakers must engage with these issues and consider the tradeoffs involved.”
Correction, June 3
The original version of this story misstated the affiliations of the researchers who contributed to the study. Two of the five researchers are affiliated with Stanford University and three with Epoch AI; they are not all with Epoch.