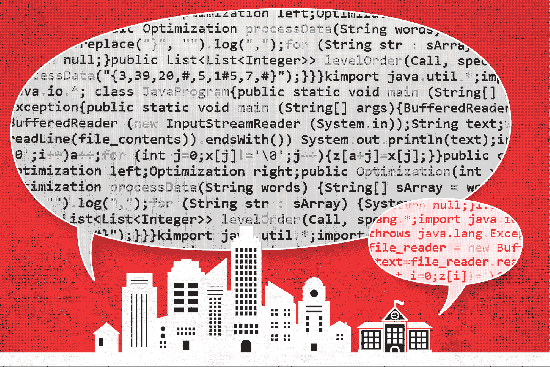
The National Artificial Intelligence Research Resource pilot could help close the gap between industry and academia.
This week, the National Science Foundation (NSF) announced it was launching a pilot program with 10 other federal agencies and 25 private sector and nonprofit organizations that could be a first step towards democratizing access to the expensive infrastructure required for cutting-edge AI research.
The National Artificial Intelligence Research Resource (NAIRR) pilot aims to provide expensive computational horsepower, datasets, AI models, and other tools to academic AI researchers who otherwise often struggle to access the resources they increasingly need.
Chipmaker Nvidia, one of the companies involved in the program, said that it would contribute $30 million worth of cloud computing resources and software to the pilot over two years, while Microsoft announced it would contribute $20 million of cloud computing credits in addition to other resources. OpenAI, Anthropic, and Meta, which are among the leading companies in the sector, are reportedly providing access to their AI models.
[time-brightcove not-tgx=”true”]The NAIRR pilot comes at a pivotal moment for AI research. As tech companies have plowed vast amounts of money into acquiring computational resources and datasets, and hiring skilled personnel, researchers in academia and the public sector have been left behind. This has resulted in crucial research directions and fundamental scientific research being left unexplored. However, commentators caution that the pilot is just an initial step, and that closing the AI divide will require sustained, ambitious government investment.
Industry pulls ahead
AI systems have three inputs—computational power (often referred to as “compute”), data, and algorithms. Greater amounts of data and compute and better-designed algorithms produce more capable AI systems. Industry’s increasingly privileged access to all three AI inputs has resulted in a widening gap between AI systems built by businesses, compared with those built by researchers in academia.
Read More: 4 Charts That Show Why AI Progress Is Unlikely to Slow Down
A couple of decades ago, the majority of exciting breakthroughs were made by researchers in academia, says Nur Ahmed, a researcher at the MIT Sloan School of Management. “Now, academics are doing more follow-up or follow-on research instead of trying to push the boundaries.”
Whereas previously the most capable AI at a given task would likely have been built by academics, now almost all cutting edge AI systems at least involved collaboration with industry, and many were entirely built by industry.

In practice, compute means access to specialized semiconductor chips, which are expensive and scarce. As access to computational power has become more economical over time, the amount used to train AI systems has been increasing steadily—doubling once every 20 months since the dawn of AI in 1950. But around 2010, as it became apparent that training models using greater amounts of compute would make them much more capable, AI developers started to train much larger models, with the amount of compute used doubling every 6 months.
Since then, the amount of money spent on training AI systems has rocketed—researchers at Epoch found compute costs have increased annually by roughly a factor of three between 2009 and 2022. Epoch data shows that academics have effectively been priced academics out of developing state of the art models.
Much of the data used to train AI systems—particularly language models, which use large amounts of data scraped from the internet—is publicly available. But industry still has two advantages over academia and the public sector, says Neil Thompson, director of MIT’s FutureTech research project.
First, wrangling the vast amounts of data used to train state-of-the-art AI models requires large amounts of compute and is made easier by having teams dedicated to data cleaning and preparation, both of which are available to industry but not academia. Second, companies often have access to proprietary datasets which are particularly valuable for their specific purposes.
Researchers design algorithms. Therefore, the organizations that can access the greatest numbers of talented people will tend to have access to the more sophisticated algorithms. In the wake of the release of ChatGPT and the subsequent artificial intelligence boom, the AI labor market is incredibly hot, says Thompson, creating intense competition for skilled researchers and engineers. Companies have been offering increasingly large salaries to attract these workers—a Netflix job posting last year offered a salary of up to $900,000. Pay gap aside, researchers are also attracted by the superior access to data and compute that industry offers, says Thompson.
This dynamic may be bad for society overall, says MIT’s Ahmed. Commercial AI developers have their own incentives, and fewer resources in academic research might mean there is less work being done on societally important issues such as addressing bias in AI systems, says Ahmed. A paper published in 2020 by researchers at the National Endowment for Science, Technology and the Arts supports Ahmed’s concerns, finding that “private sector AI researchers tend to specialize in data and computationally intensive deep learning methods at the expense of… research that considers the societal and ethical implications of AI or applies it in domains like health.”
Left to their own devices, private actors tend to underfund basic research, says Thompson. And without sufficient compute, academics and public sector researchers won’t even be able to check the work of industrial researchers.
Closing the divide
The pilot announced this week has been a long time in the making. The NAIRR Act, passed in 2020, established a task force to develop a roadmap for a national program to improve access to computing, data, and educational tools. The NAIRR Task Force’s final report, published in January 2023, said that $2.6 billion would be required to operate the NAIRR over six years, and suggested a pilot as a way of moving forward in the absence of the full funding. President Biden’s AI Executive Order, signed Oct. 30, gave the NSF 90 days—until Jan. 28—to launch a NAIRR pilot.
The pilot, though welcome, is not sufficient, says Divyansh Kaushik, associate director for emerging technologies and national security at the Federation of American Scientists, who advised the NAIRR Task Force. Congress must pass laws authorizing the NAIRR and making available the funds required, he says, adding that most lawmakers are in favor of the program. “There’s not really any opposition,” he says.
Such a law was proposed in July, when the leadership of the Congressional Artificial Intelligence Caucus introduced the CREATE AI Act, which would establish the NAIRR. Senators Martin Heinrich, Todd Young, Cory Booker, and Mike Rounds introduced a companion bill in the Senate. “We stuck pretty much to do what the task force recommended. In my view, they did very good work,” Congresswoman Anna Eshoo, a California Democrat and a co-chair of the Congressional Artificial Intelligence Caucus, told TIME in September 2023.
“The NAIRR will provide researchers—from universities, nonprofits, from government—with the powerful data sets and computing resources that are really necessary,” said Eshoo. “To ensure that everyone has access to the tools that are needed for the research and development of AI systems that are safe, that are ethical, that are transparent, and that are inclusive.”
In addition to the NAIRR Act, lawmakers should take steps to expand the government’s access to computing power, says Kaushik. This could involve building new government supercomputers in line with a U.S. Department of Energy report released in May that has the backing of West Virginia Democrat Senator Joe Manchin, he suggests.
“NAIRR is an incredibly important first step, but it’s just the first step. That’s not going to be enough to meet the demand for all the public sector stuff, and the publicly minded stuff that academics should be doing and would want to do,” says Thompson of MIT. “We’re just going to need to keep investing to get more and more scale here.”